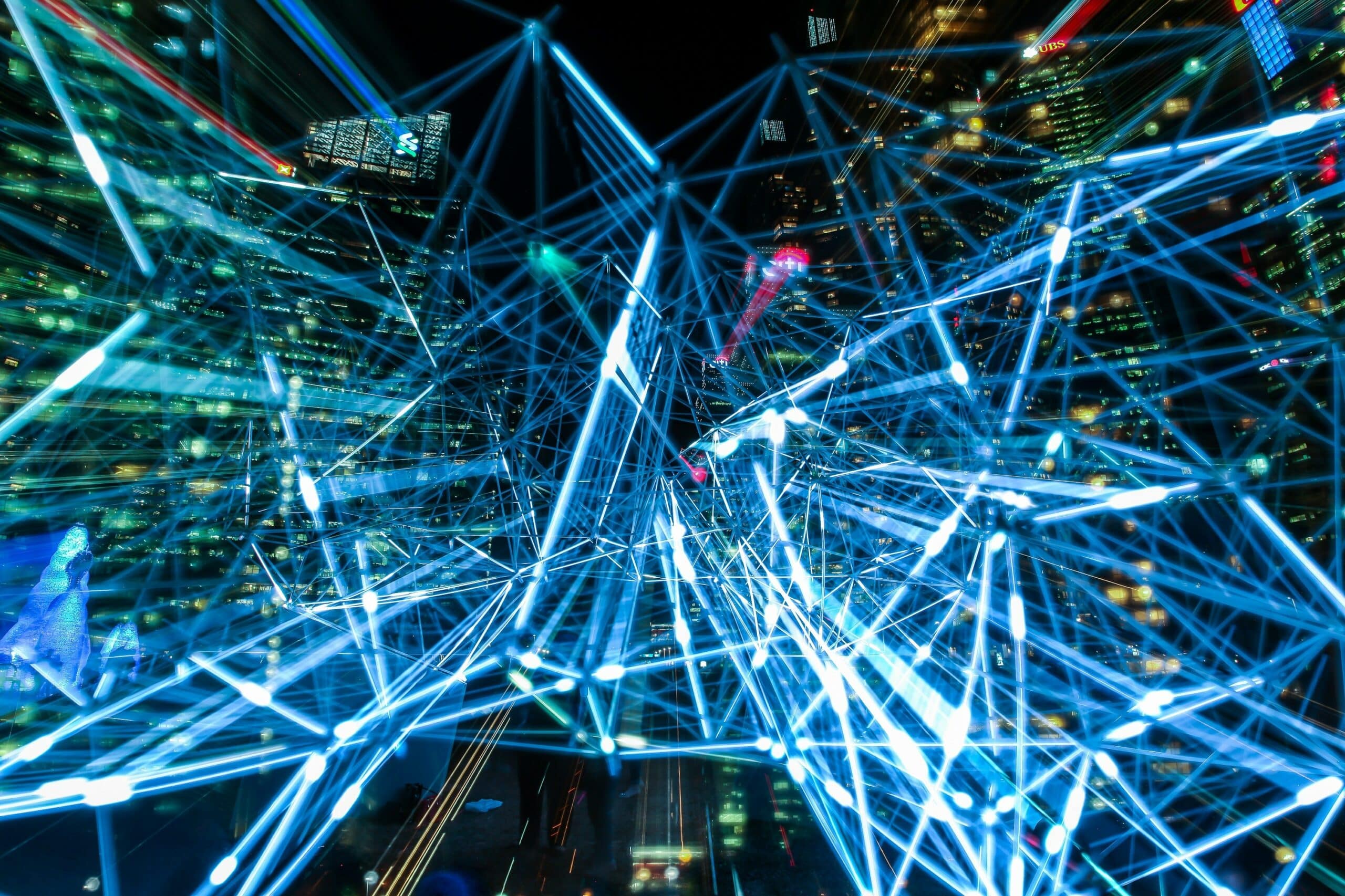
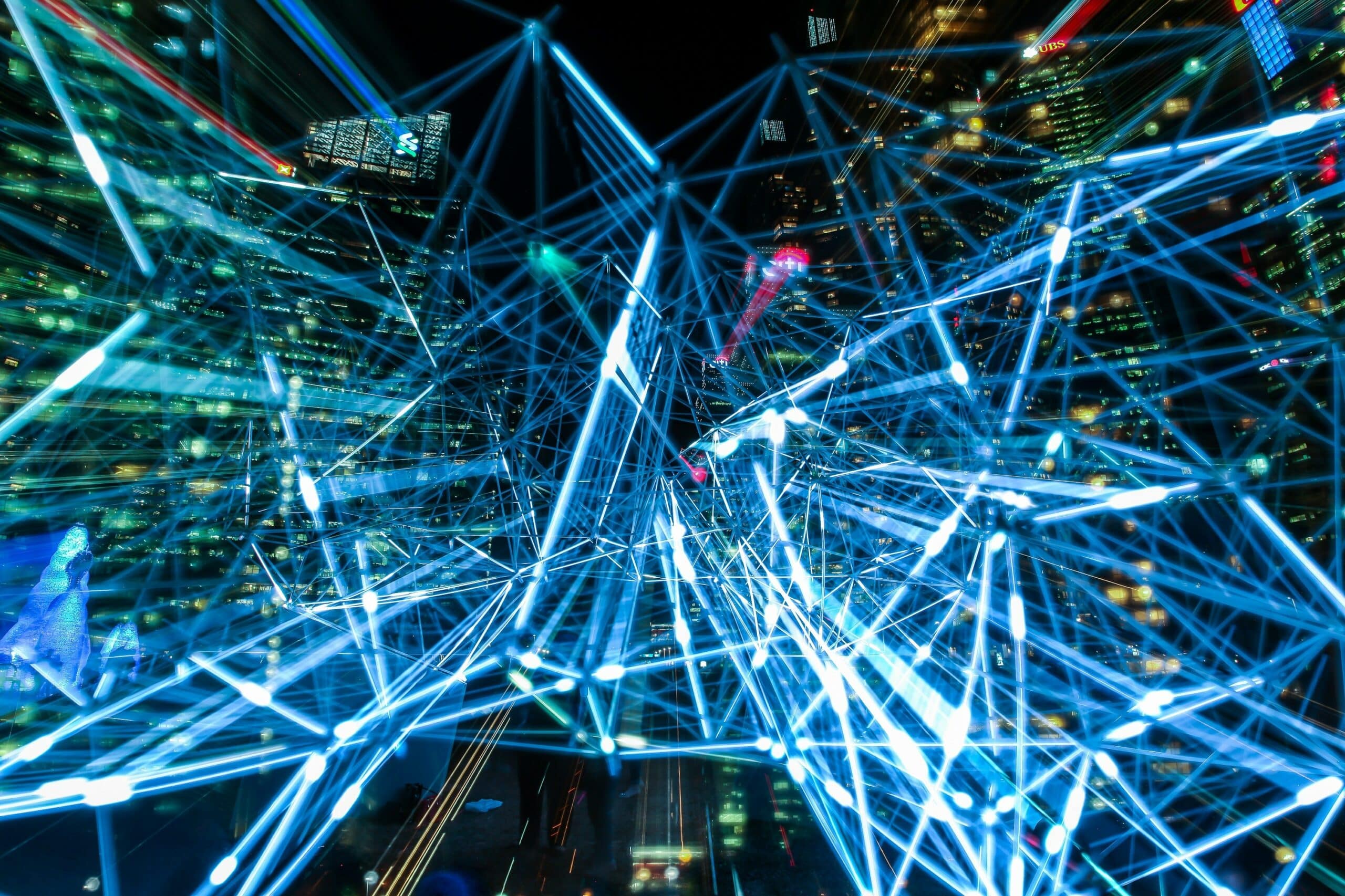
With her funky orange hair and winning smile, Aimie is an instantly recognizable avatar to anyone familiar with Sidetrade. She’s the personification of our AI technology, and acts as a digital assistant – an extra member of the team – to Sidetrade customers in over 80 countries.
We know that Aimie is a valuable addition to any collections team, making their lives easier through intelligent automation and improving overall efficiency of collections efforts. But with the term ‘artificial intelligence’ being used as shorthand to describe many other modern software solutions in the Accounts Receivable space, we wanted to define exactly what ‘artificial intelligence’ means to us – including how Aimie learns, how she generates actions, how we measure her success, and why we’re confident she’s the best digital assistant out there.
Over the last 3 years alone, that has grown by more than 330m payment behaviors and over €953bn (US$1trillion) in B2B transactions
Our data
Situated at the core of any AI system, data plays a vital role in everything from how the AI learns to the types of decisions the AI can make. For Aimie, it all begins with our datalake, which comprises over 20 years of customer payment behavior data across a range of industry sectors. Over the last 3 years alone, that has grown by more than 330m payment behaviors and over €953bn (US$1trillion) in B2B transactions. When predicting the payment actions of a customer, Aimie considers a range of data points in four main categories:
- Invoice information (data points include amount, payment methods and item type)
- Company information (for data points such as revenue, net income, industry and vendor currency)
- Previous action information (data points include action type, number of items dunned in the action, and day of the week the action was executed)
- Current action information (with data points similar to the previous, and also including granted payment term, number of days since the last action was executed, and delay of the leading invoice at the time of the action).
As long as we have a certain number of data points in a particular sector, Aimie can extrapolate this data to predict the ‘best next action’ for a collector to take. That way, the minute one of our clients implements Aimie, she’s adding value by using her existing knowledge to intelligently predict the best fit dunning actions for their customers. From that moment on, Aimie then takes each customer interaction – plus our client’s own historical customer payment data – into account when recommending actions or automating tasks, which perpetuates the cycle of continuous learning and ensures she’s always using the most relevant data points to match customers with collection recommendations.
Aimie success metrics
Being able to measure Aimie’s effectiveness is crucial to our ongoing development projects – but it also provides us with concrete statistics about her performance in relation to her human counterparts. In a previous blog post we shared some figures that show how Aimie often outperformed human collectors in terms of efficiency throughout the Covid-19 crisis, and having this data to hand means we can be confident in Aimie’s abilities.
We measure Aimie’s efficiency by counting the days it takes for payment to arrive following an action being completed. If payment arrives within 7 days, we class this as an ‘efficient action’. Using this metric, we’re able to compare the efficiency of Aimie to the efficiency of human collectors following a workflow. Recently, we analyzed Aimie’s performance throughout the pandemic, and found that one of our clients was able to entirely automate their dunning and collections process, increasing the efficiency of actions to 68% and reducing the need for manual intervention while their collections team was furloughed. Not only does this prove Aimie’s success, it also shows us that digital assistants powered by machine learning algorithms, like Aimie, can adapt even when faced with unprecedented challenges.
According to figures from Pymnts.com, the average DSO with no or low-level tech is 52 days, which dramatically reduces to 40 days with high-level automation in place. Adopt Sidetrade’s order-to-cash platform and welcome Aimie into your team – she’ll act as a virtual assistant to prioritize workload, improve the efficiency of your dunning process through automation, give you more insight into your buyers’ behavior, and help you benefit from improved working capital and reduced DSO. Aimie is the assistant you never knew you needed, but once you start working with her, you’ll wonder how you ever managed without her!